OpenAI's o1: A Game Changer for AI and AGI?
Meta Description: OpenAI's o1, a new AI model with enhanced reasoning capabilities, has sparked debate about its impact on the future of AGI and the Chinese AI landscape. Explore the potential benefits and challenges of this new paradigm shift.
The emergence of OpenAI's o1 has sent shockwaves through the AI community, challenging the traditional paradigm and sparking a new wave of excitement and speculation. This innovative model, distinct from its predecessors in the GPT series, utilizes reinforcement learning to achieve a level of reasoning prowess previously unseen in large language models. This breakthrough has ignited a heated discussion: will o1 reshape the trajectory of AI development, particularly in China?
The o1 Revolution: A Paradigm Shift in AI
OpenAI's o1 is not just another incremental improvement; it's a significant leap forward in AI development. By integrating reinforcement learning, o1 demonstrates a remarkable ability to engage in "slow thinking," a key attribute of human intelligence. This allows it to actively reflect, refine its reasoning, and correct errors, demonstrating a level of cognitive complexity previously unheard of in AI.
Beyond Scaling: Breaking the Data Wall
The traditional approach to AI advancement has heavily relied on the "Scaling Law," focusing primarily on increasing model size and data volume. However, o1's introduction signifies a departure from this paradigm. By incorporating reinforcement learning and introducing innovative techniques like "thought chains," o1 effectively addresses the limitations of the "data wall" and unlocks new avenues for AI progress.
A New Era of AI: The Convergence of System 1 and System 2
o1's ability to leverage both "System 1" (fast thinking, providing immediate answers) and "System 2" (slow thinking, engaging in complex reasoning) makes it a unique entity in the AI landscape. This dual capability sets o1 apart, marking a critical milestone in AI development. It's a testament to the model's ability to emulate the intricate processes of human cognition, paving the way for more sophisticated and nuanced AI systems.
The Road to AGI: A Multi-faceted Journey
Despite concerns that AGI development is slowing down, experts like Jiang Daxin argue that the opposite is true. He proposes a three-phase approach to achieving AGI: simulating the world, exploring the world, and abstracting the world. o1 represents a significant leap forward in the abstraction phase, while GPT-4o demonstrates progress in simulating the world through multi-modal fusion. Meanwhile, Tesla's FSD V12 system provides insights into how embodied AI can interact with the physical world, pushing the boundaries of exploration.
The Impact on China's AI Landscape
The emergence of o1 has a profound impact on China's AI development. While China has made significant strides in AI research and development, o1 represents a new benchmark and a challenge to existing strategies. The integration of reinforcement learning into large language models could fundamentally alter the landscape, prompting a reassessment of current approaches and fostering new avenues for innovation.
The Opportunities and Challenges of o1's New Paradigm
o1's success raises several crucial questions: How can we effectively generalize its capabilities beyond specific domains? What are the implications for the training and inference of future models? And how can we ensure that o1’s advancements translate into real-world applications?
The Importance of Process Supervision
While o1's technical implementation remains undisclosed, experts like Zhu Jun emphasize the critical role of "process supervision" in achieving generalization. Unlike traditional result-oriented supervision, process supervision involves annotating every step of the reasoning process, demanding significant expertise and resources. This aspect presents both a challenge and an opportunity for future research and development.
The Role of Reward Models
Defining effective "reward models" is critical for navigating the challenges of generalization in reinforcement learning. Reward models, which guide the learning process by evaluating an AI agent's actions, become increasingly complex as AI systems venture into more open and dynamic environments. Addressing this challenge is key to unlocking the full potential of o1's new paradigm.
A New Landscape for AI Entrepreneurs
o1's emergence creates both challenges and opportunities for AI startups. The shift towards reinforcement learning necessitates a reevaluation of the balance between training and inference. This change could empower startups to focus on foundational AI innovation, developing algorithms that optimize training and inference processes. Furthermore, startups can leverage the power of o1 and similar models to achieve specific outcomes in various sectors, leveraging the "fine-tuning" capabilities of these advanced models.
The Future of AI: A Continuous Quest for Innovation
The success of o1 signifies a new era of AI development, characterized by a relentless pursuit of innovation. As we delve deeper into the intricacies of reinforcement learning and its integration with large language models, we can expect to witness groundbreaking advancements in AI capabilities, pushing the boundaries of what was once thought impossible. This journey will undoubtedly be filled with challenges, but it also holds the promise of transformative breakthroughs that could reshape our world in profound ways.
The Power of Reinforcement Learning
H2: Unlocking the Potential of Reinforcement Learning
Reinforcement learning (RL) has emerged as a powerful tool in the arsenal of AI, offering new possibilities for developing intelligent agents that can learn and adapt in complex environments. This approach involves training an agent to make decisions based on its interactions with the environment, guided by a system of rewards and penalties.
The Key Features of Reinforcement Learning:
- Interactive Learning: RL agents learn through continuous interaction with the environment, receiving feedback in the form of rewards or penalties for their actions. This iterative process allows the agent to refine its decision-making strategies over time, ultimately maximizing its rewards.
- Goal-Oriented Learning: RL agents are trained to achieve specific goals or objectives, such as navigating a maze, playing a game, or completing a task. The agent's learning process is driven by its desire to maximize its rewards and achieve its desired outcome.
- Adaptive Learning: RL agents are capable of adapting to changing environments and learning new strategies in response to unexpected challenges. This adaptability makes RL particularly well-suited for real-world applications where environments are often complex and dynamic.
The Benefits of Reinforcement Learning:
- Improved Decision-Making: RL excels at optimizing decision-making processes in complex environments where traditional methods may fall short. By learning from experience and adapting to new situations, RL agents can make informed decisions that lead to optimal outcomes.
- Enhanced Efficiency: RL can optimize resource utilization and streamline complex processes by identifying the most efficient strategies for achieving specific goals. This can lead to significant cost savings and improved performance in various domains.
- Autonomous Learning: RL empowers AI agents to learn autonomously, without explicit programming or human intervention. This allows for the development of intelligent systems that can adapt and evolve in response to changing circumstances, making them more robust and flexible.
The Challenges of Reinforcement Learning:
- Data Requirements: RL often requires vast amounts of data to effectively train agents. Gathering and annotating this data can be time-consuming and expensive, posing a significant challenge for researchers and developers.
- Generalization: A key challenge in RL is achieving generalization, allowing trained agents to perform well in new environments or scenarios that differ from the training data. This is a critical factor in developing agents that can effectively adapt to real-world complexities.
- Reward Model Design: Designing appropriate reward models is crucial for guiding RL agents towards desired outcomes. Developing accurate and effective reward models is essential for ensuring that agents learn to perform the desired tasks effectively.
The Future of Reinforcement Learning:
Despite the challenges, reinforcement learning is poised to play an increasingly important role in the future of AI. As researchers and engineers continue to develop new techniques and address existing challenges, RL will become even more powerful and versatile, driving innovation in a wide range of applications.
FAQs about OpenAI's o1 and its Implications
Q1: What are the key differences between o1 and previous GPT models?
A1: The most significant difference lies in o1's incorporation of reinforcement learning. This allows it to engage in "slow thinking," enabling the model to reason, reflect, and correct errors in a way that previous GPT models couldn't. Furthermore, o1 has demonstrated the ability to combine "System 1" (fast thinking) and "System 2" (slow thinking), mimicking human cognitive processes more closely.
Q2: How does o1 address the limitations of the "data wall" in AI development?
A2: The "data wall" refers to the limitations of traditional AI approaches, which rely heavily on massive amounts of data. o1's use of reinforcement learning and innovative techniques like "thought chains" allows it to achieve significant progress without solely depending on massive datasets. This effectively breaks the "data wall," opening up new avenues for AI development.
Q3: What are the potential implications of o1 for the Chinese AI landscape?
A3: o1 represents a paradigm shift in AI development, challenging existing strategies and prompting a reassessment of traditional approaches. China's AI community will need to adapt to this new landscape, potentially leading to increased investment in reinforcement learning research and development.
Q4: What are the key challenges in generalizing o1's capabilities?
A4: Generalizing o1's abilities to new domains requires overcoming several challenges. One critical aspect is the need for "process supervision," which involves annotating every step of the reasoning process. This demands significant expertise and resources, presenting a substantial hurdle. Additionally, designing effective "reward models" for open and dynamic environments is essential for ensuring that o1's learning process remains aligned with desired outcomes.
Q5: What are the potential opportunities for AI startups in the wake of o1's emergence?
A5: The shift towards reinforcement learning creates opportunities for startups focusing on foundational AI innovation. They can develop algorithms that optimize training and inference processes, leveraging the power of o1 and similar models to achieve specific outcomes in various sectors. Furthermore, startups can focus on fine-tuning pre-trained models to achieve specific goals within their respective domains.
Q6: What are the potential ethical considerations surrounding the development of AI models like o1?
A6: The increasing sophistication of AI models like o1 raises ethical concerns regarding bias, transparency, and accountability. It's crucial to develop robust ethical frameworks and guidelines to ensure responsible development and deployment of these technologies. Furthermore, addressing potential societal impacts, such as job displacement and potential misuse, is paramount in shaping the ethical landscape of AI development.
Conclusion: A New Chapter in the AI Story
OpenAI's o1 is a powerful testament to the relentless progress in AI development. This innovative model marks a significant departure from traditional paradigms, introducing reinforcement learning as a driving force in AI advancement. The potential implications are vast, ranging from reshaping the AI landscape to fostering new opportunities for entrepreneurs and researchers.
However, this journey is not without its challenges. Generalizing o1's capabilities beyond specific domains requires addressing thorny issues related to data, reward models, and ethical considerations. Nevertheless, the promise of AI systems that can reason, reflect, and adapt like humans is undeniably exciting.
As we continue to explore the possibilities of AI models like o1, we must foster a collaborative and responsible approach to its development and deployment. Only through a concerted effort from researchers, developers, policymakers, and the public can we harness the power of AI to create a brighter and more equitable future for all.
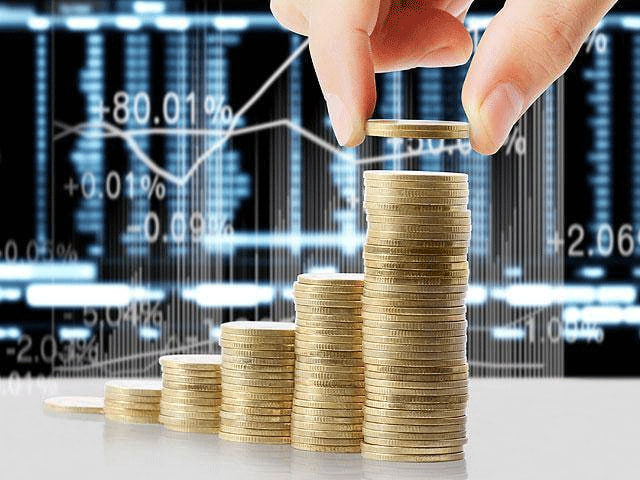